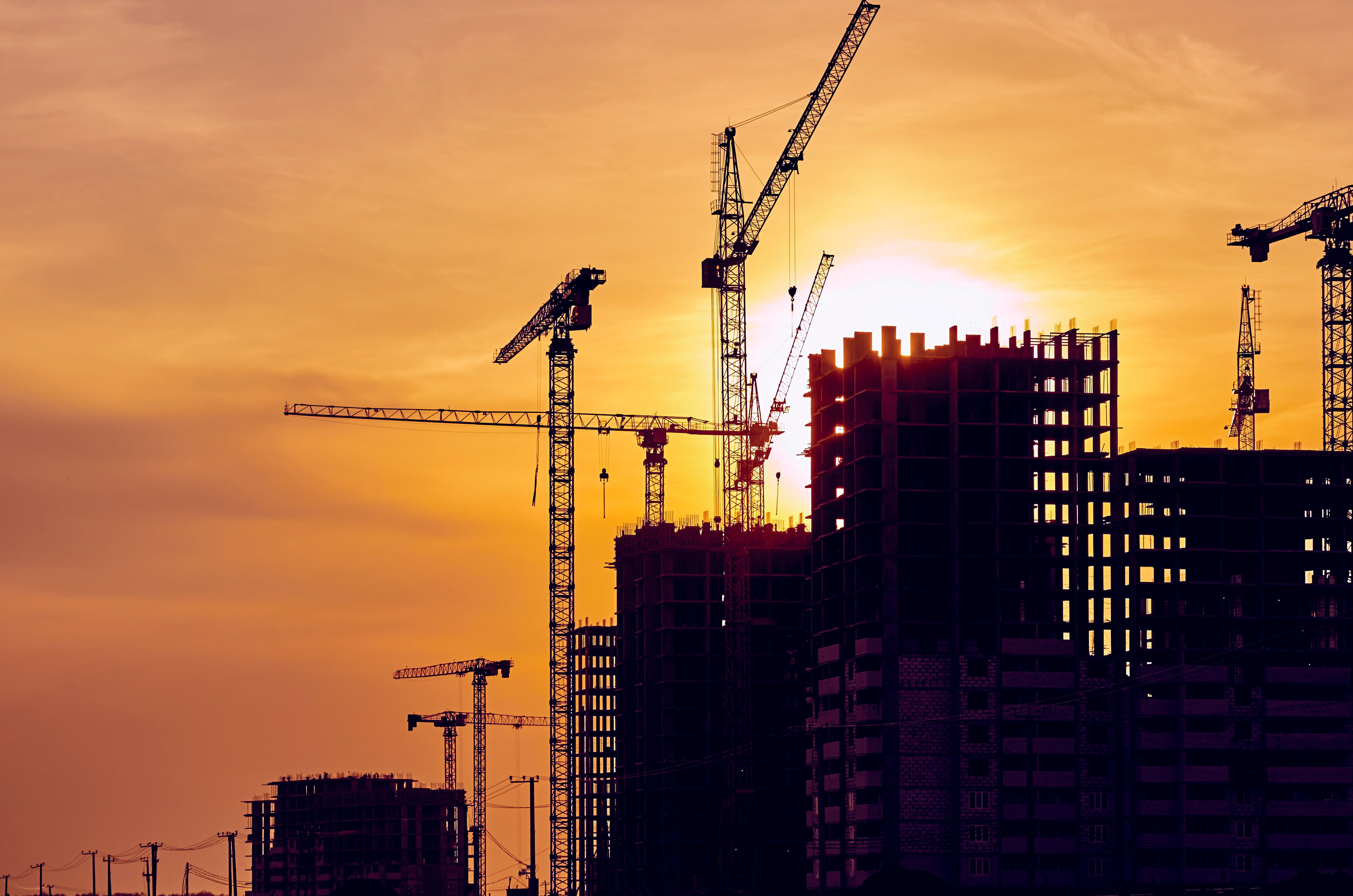
The rise of machine learning: the risks
In last week’s article of our generative AI mini-series, we provided some information about how Large Language Models (LLM’s) work. In the reflections, we identified concerns about the way LLM's self-learn and material being shared with open-source applications.
This article, part 2, explores the risks further and considers how those risks can be managed. The article concludes with considerations as to what is next for LLM's.
What are the risks?
The most obvious risk is the LLM’s ability to train itself, without human prompt, meaning the content cannot be fully verified before it is relied upon. An example would be two conflicting data sets and the LLM deciding which it considers to be the most authoritative. Particularly in a business setting, where the stakes are often high, this is concerning and raises questions about the accuracy of content created which is drawn from internet information contaminated with fake news.
The risks may go further. What if the LLM single-mindedly pursued a rigid instruction set by a human that causes unintentional harm? As a hypothetical example, Nick Bostrom described an experiment, called the “paperclip maximiser”, where an instruction is given to manufacture as many paper clips as possible and the machine takes any measure necessary to cover the Earth in paper-clip factories.
A wild theory, but the example illustrates the dangers.
In the short term, internet harm perpetuating today will be accelerated with the use of LLM’s because they are ideal, if used with ill-intent, for spreading misinformation, scamming people out of money or convincing people to click malware infected emails. Again, in the wrong hands, the use of LLM’s to make new discoveries in science mean it is conceivable that they could be used to produce drugs or exploding devices.
How are the risks being managed?
The risks are being addressed and OpenAI, ChatGP4’s developer, has used several approaches to reduce the risk of accidents and misuse. One approach is to use “reinforcement learning from human feedback”, where humans provide feedback on the appropriateness of a response. Another is “red teaming”, where the model is attacked by trying to get it to do something it shout not do. This is an attempt to get ahead of “jailbreakers” who have put together websites littered with techniques for getting around the model’s guardrails.
There are suggestions that AI needs to police AI to assess whether its responses adhere to constitutional principles. However, this raises questions about who writes the constitution, and it would require alignment between powerful countries: a possibility which seems unlikely given the unstable geopolitical landscape. The possibility of cyber-attacks mean that the AI could, unknown to the user, be changed to generate content that suits the cyber attackers’ motives.
What next for LLM’s
Their rate of development has been astonishing and LLM’s are increasingly being embedded in both day-today life and business life.
There are some think that LLM’s in their current form are doomed and that efforts to control their output or prevent them from making factual errors will fail. They believe that LLM’s have taken too many wrong turns and are an “off-ramp” away from the road towards a more powerful AI. This may, or may not be the case, and only exploration and testing of the AI technology will determine whether LLM’s, in their current form, will stand the test of time.
However, I consider it is only a matter of time before they are as prevalent in our lives as email or social media. It is likely that people will explore the technology and find new and exciting ways to improve their lives and / or the productivity and performance of their businesses. This exploration means there will be successes and failures, and the development of LLM’s is dependent on both. It seems plausible that, through this process, someone will find a way to scale an LLM for a large group of people (e.g., a large company or a whole industry).
This crucible moment is probably not as far away as it seems, and the opportunity for the construction industry is ripe. We are the worst performing industry in the UK when it comes to productivity and suffer from a chronic, and never ending, skills shortage. We are an industry which has a rich abundance of data and systems but lacks the wherewithal to yield quick and effective performance insights.
Final Reflections
Whilst there are undoubtedly risks associated with the use of LLM’s, and organisations need to educate their people about these risks, there are unquantifiable opportunities. We struggle in the construction industry to process large data sets and LLM’s provide a solution to this challenge.
The most exciting aspect for me is the ease of the interface. You don’t need to be a tech whizz to use these tools. They are accessible to anyone in the industry who has a basic understanding of how computers work, something that most either learn through school or through day-to-day use of technology in their lives.
The question is whether we, as a construction industry, can learn to harness the technology, or will we start to see tech companies move in and disrupt construction as they have done in so many other industries?
In next week’s article, we will move on to explore the opportunities further.
Credit The Economist
https://www.economist.com/science-and-technology/2023/04/19/how-generative-models-could-go-wrong
Back to insights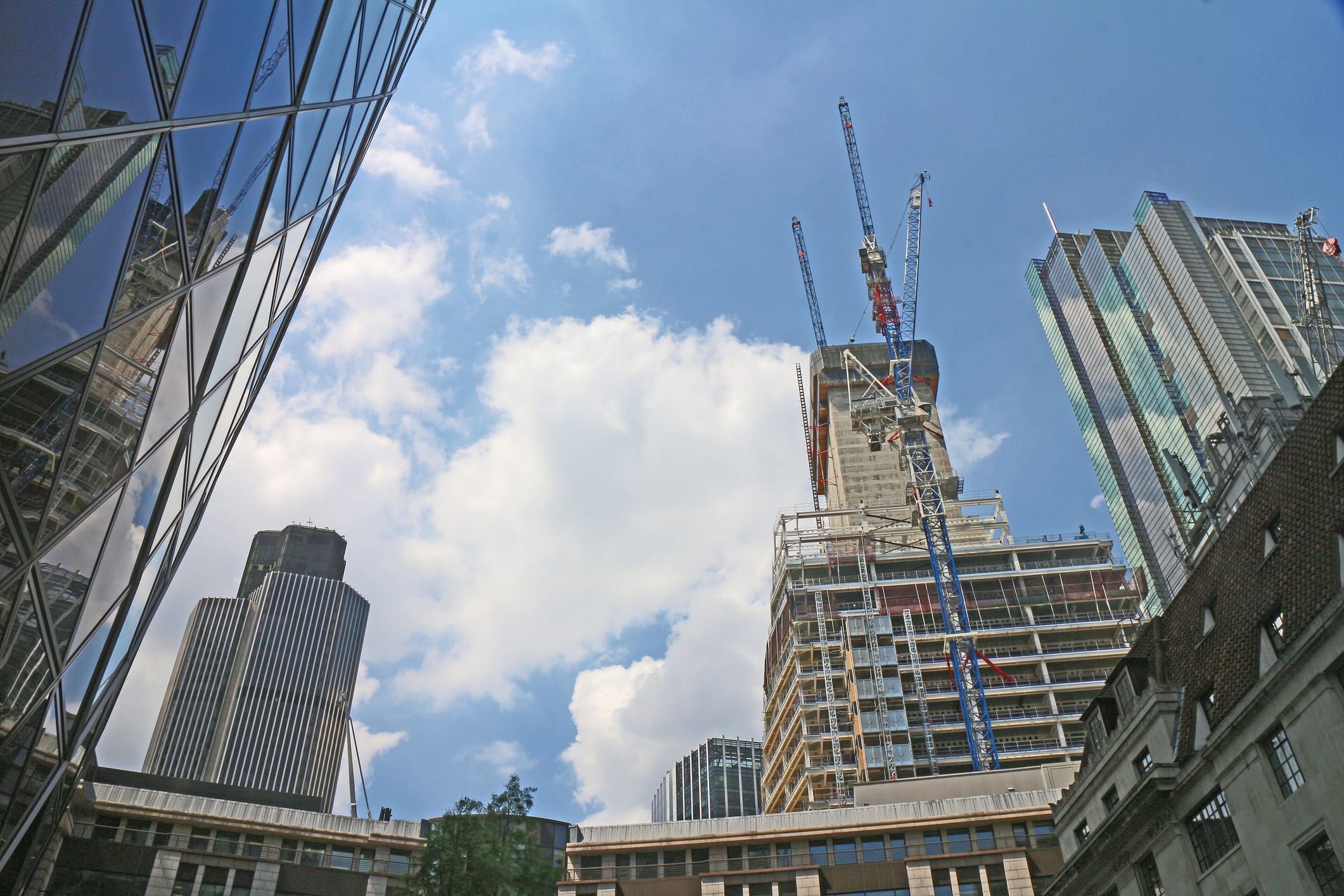
THE SCIENCE OF QUANTIK™
Publications
We publish insights through our LinkedIn newsletter, titled “The science of Quantik”, which are light bites of information covering news and insights relating to the construction industry and quantity surveying.
LinkedIn