
The rise of machine learning: how generative AI works
On the back of our construction productivity and #thefutureofquantitysurveying mini-series, we move into the next mini-series focused on generative AI.
As QS’s, like all professions, we will increasingly use generative AI in our day-to-day work and lives so, in using them, we should seek to understand how they work.
Whilst there is a lot of talk about generative AI, I found there was very little in the way of information about what they are, how they work, and what are the limitations / risks.
The purpose of this generative AI mini-series is to summarise research I have undertaken in a concise and easy-to-understand way. This first article in the mini-series focuses on how generative AI, referred to as Large Language Models, work and will lead into future articles about both the risks and the opportunities to the quantity surveying profession.
Introduction
Are you excitedly redesigning your work methods ready to ride the ChatGPT wave, bunkering down ready for the existential threat, or simply sat waiting to see how all the drama unfolds? Whichever approach you are taking, one thing is for sure: it is inevitable that Large Language Models (LLM’s), such as ChatGPT, will reshape how we work and live.
The astonishing rate at which LLM’s have developed has taken many by surprise. They embody more knowledge than a human has ever known and perform tasks that had been exclusively in the domain of human intelligence.
Here are some facts which signify the scale of their development:
- GPT-3.5 flunked the American Uniform Bar Examination whereas GPT4 passed in the 90th percentile.
- GPT-3 can process 2,048 tokens whereas GPT-4 can process up to 32,000 tokens.
- The first version of GPT has one-ten-thousandth of the size of GPT-3’s hundreds of layers, billions of weights and training using hundreds of billions of words.
This development rate has raised concerns among prominent voices, including Elon Musk, that LLM’s need to be paused because the capabilities have outrun the understanding and control of their creators. These concerns have raised questions about the impact of LLM’s but, so far, their development has not been paused and there are new possibilities arising every day.
What are LLM’s and how do they work?
An LLM is an artificial intelligence (AI) which uses deep learning techniques and large data sets to understand, summarize, generate, and predict new content. The LLM understands language in a statistical way, which differs from humans who understand language grammatically. LLM’s are a development of deep learning but, unlike deep learning, they can be more easily used by people who do not have coding skills; this opens the possibility of using LLM’s on a mass scale.
The LLM works in the following way:
- A written query is converted from words into numbers and the text is split into tokens (chunks of characters).
- The tokens are embedded into a meaning space where words, with similar meanings, are located in nearby areas.
- Attention networks are deployed to make connections between different parts of the prompt.
- The LLM repeats a process called autoregression where a word is generated and the LLM feeds the result back into itself; this is repeated until the LLM has finished.
- A response is initiated when the prompt has been processed and the attention network has produced a probability of that token being the most appropriate one to use next in the sentence it is generating.
The initial concept of the LLM might be developed by a human but it's self-learning, without human prompt, means that a human would not know, with any precision, how the LLM works.
The LLM’s self-learning approach involves quizzing itself on a chunk of text it is given, covering up the words at the end, and trying to guess what might go there. The answer is uncovered and compares this to its guess. A loss is generated and sent back into the neural network to nudge the weights in a direction that will produce better answers.
What are the limitations of LLM’s?
Whilst the rate of development has been exponential it appears unlikely that this progress will continue an indefinite straight line, with some saying that GPT-4 may have reached an inflection point. It is considered that the following issues will limit the development and use of LLM’s:
- Data availability: there is only so much data available and the stock of high-quality language data, available on the internet, will soon be exhausted.
- Investment: training GPT-3 cost OpenAI an estimated $4.6m whereas GPT-4 cost disproportionately more, circa $100m, meaning advancements will require even more investment.
- Computational power: no new hardware is forthcoming which offers a leap in performance as large as that which came from using GPUs in the early 2010s so training larger models will become increasingly expensive.
- Chip manufacture: this is not increasing exponentially, and this will limit how fast LLM’s can improve.
- Legal issues: the LLM uses data that is often copyright material being used without permission and, whilst there might be a fair use argument, this issue will inevitably be tested in court one day.
Final Reflections
The article has provided a bite size introduction into how LLM’s work and identified some of the limitations.
The aspect that spoke to me most as a QS was the way LLM’s self-learn. You may understand the question you have asked and the answer you have been given, but you would not be able to trace the route from one to the other.
A further aspect which provoked a thought in my mind was ownership of material . I expect this will increasingly become an issue, and we need to be careful about what material is given to open-source applications. If you are sharing commercially sensitive information, then you may need to rethink this and make sure the relevant permission.
In next week's article, the risks associated with LLM's will be explored further.
Note: This is a refresh of an article I wrote in 2023.
Credit The Economist:
https://www.economist.com/science-and-technology/2023/04/19/how-generative-models-could-go-wrong
Back to insights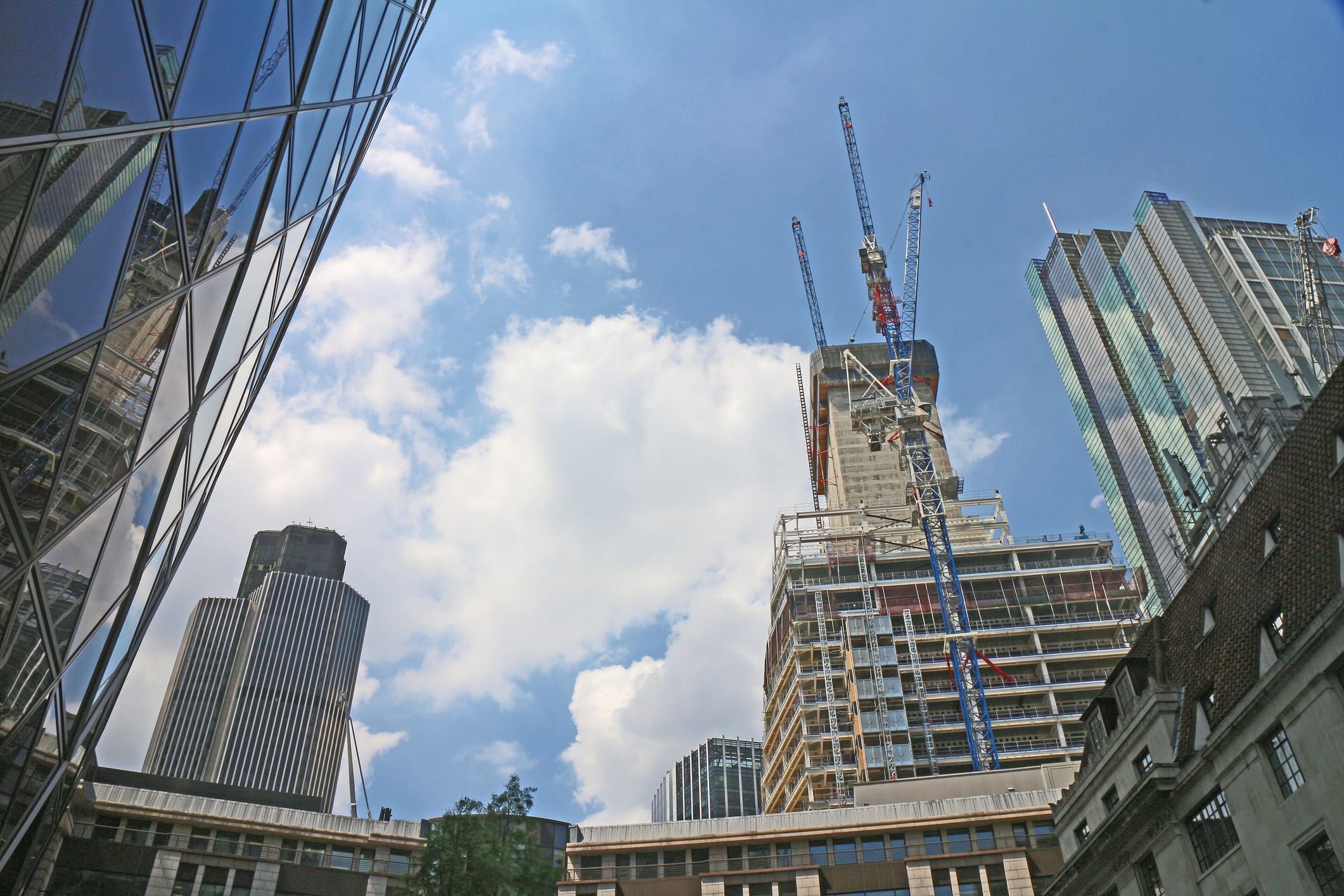
THE SCIENCE OF QUANTIK™
Publications
We publish insights through our LinkedIn newsletter, titled “The science of Quantik”, which are light bites of information covering news and insights relating to the construction industry and quantity surveying.
LinkedIn