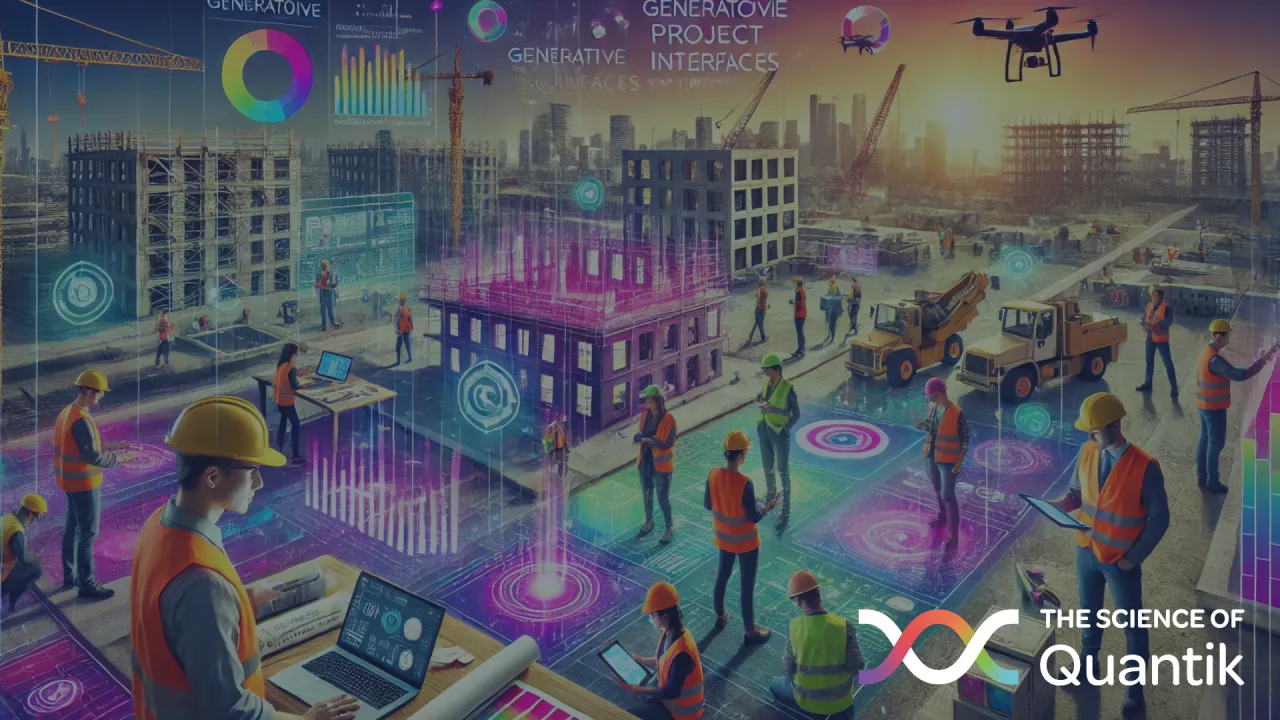
Quantity Surveying x AI: barriers and benefits
In last week’s article we introduced this mini-series, which will explore issues and challenges relating to quantity surveying and the constantly evolving issue of generative AI and explore the productivity benefits generative will bring and the benefits this could have on the UK’s ability to build more for less.
The conclusion of the article, which is the subject of this week’s article, is that, despite the obvious benefits of generative AI, implementation in the profession is relatively slow, leading to a question as to whether there are too many barriers (the risk is too great) or the benefits are not fully understood (the opportunity is not big enough).
Working smarter and not harder
We work in an industry that is, by its nature, always looking to work harder rather than smarter. There is a natural resistance to change because people are overwhelmed with work to do, and there is very little space in the weekly work plan for creative thinking or testing new ideas.
These generative AI tools cannot be implemented as an off-the-shelf solution, so if they are to be adopted effectively, then time is needed to work out which applications are the most suitable and what information is needed to generate the output required. Why would a QS prioritise this time investment when the Excel sheet, despite being labour intensive every month, gets you to the answer?
It’s a cultural issue and will need enlightened and strong leadership to move a QS team towards new possibilities with the psychological safety to try, fail, and try again. In an industry, and a profession, that does not traditionally embrace technological change with open arms and tends to have a strong preference for Excel, it is difficult to see a transition towards working smarter rather than harder.
Let's wait and see
It is most likely that most in the industry will adopt a ‘let’s wait and see’ approach; they will wait for someone to trailblaze and then look to adopt those ideas. The QS in me sees some sense in this. It is a lot harder, and therefore more time intensive and costly, to forge a new path than to follow others down it.
One would hope that young professionals and those entering the industry will adopt a way of working that is more modern and naturally uses digital tools and applications to get things done. Over time, there is a possibility that could challenge normal convention and lead to gradual improvement in the way things are done. That may always lag the development of generative AI technology, and there will be some that say, quite sensibly, that it isn’t a bad thing.
This would be improved if RICS-accredited courses and the assessment of professional competence (APC) criteria placed a much greater emphasis on data science skills. The data management competency has always been a mandatory level 1 competency, as far as I can recall, but knowing about data management is nowhere near enough to prepare a young professional for the world of work, let alone advance the profession.
I feel wait and see will probably be the approach of the majority, but if skills are not developed, then you have to wonder how long this approach will take before we see noticeable advances in the way we work.
Data, data, and more data...but no structure
We still haven’t fixed the problem that existed ten years ago, and there is a possibility that it will still exist in ten years. Despite being a data-rich industry with systems upon systems in most businesses, we have always been information-poor.
How many times have you been in a meeting where someone asks what seems to be a simple question, and the answer is not simple because the process of obtaining the pieces of information is very convoluted? You watch on, becoming dizzier by the second, while someone jumps from spreadsheet to spreadsheet and, in the end, either forgets the question or answers a completely different one.
The absence of structure in the way data is entered and captured is a major problem in the construction industry. There is often an absence of ‘system thinking’ where someone sets up a process to capture data (e.g. a site record) but does not think about what insights are needed from the data and how that data can be analysed to deliver those insights.
The situation we find ourselves in is nobody’s ‘fault’. We are people who entered a profession that involved engineering, law and finance-type issues only to become DIY data scientists. Whilst the current situation is, to a large extent, what it is, what we can do is improve the way insights are yielded from data by improving the way we structure and capture data.
Policies and privacy
The RICS public consultation on a new professional standard for responsible AI use closed on 29 April 2025. The subject of the consultation is the first edition of a professional standard which establishes the standard expected of all RICS surveyors.
I can see the need for this professional standard. When you receive a document prepared by an RICS surveyor, how do you know that it has been prepared by that person and isn’t the output of a generative AI tool? You would like to think it doesn’t happen, but, sadly, it almost definitely does.
If there is no professional standard, then, whilst using generative AI and passing it off as your work might be viewed dimly, there is no guidance against which to test whether conduct is deemed to be professional or unprofessional.
If a surveyor is using generative AI, then do they understand what they are putting into these applications and how applications use that information? Do they even have permission to use the information in the way that they have, and if they don’t, then what is the remedy for the client whose information has unintentionally become available to others?
I like the idea that surveyors should disclose whether, and how, they have used generative AI in their work. In my view this should be the norm – for the record, this article is entirely my own work!
Final reflections
Whilst the use case for generative AI is easy to make, there are numerous people and policy barriers to its implementation. I don’t see that slowing down the technology, but I do see it slowing down implementation.
This slow and steady, cautious approach of those with a conservative approach might frustrate the progressives among us, but I can see the logic. We need to know how the information is being used, implement and develop the professional standards for its use, and probably focus on the more pressing challenge of structuring our data in a way that will enable generative AI to work effectively for those in the profession.
Back to articles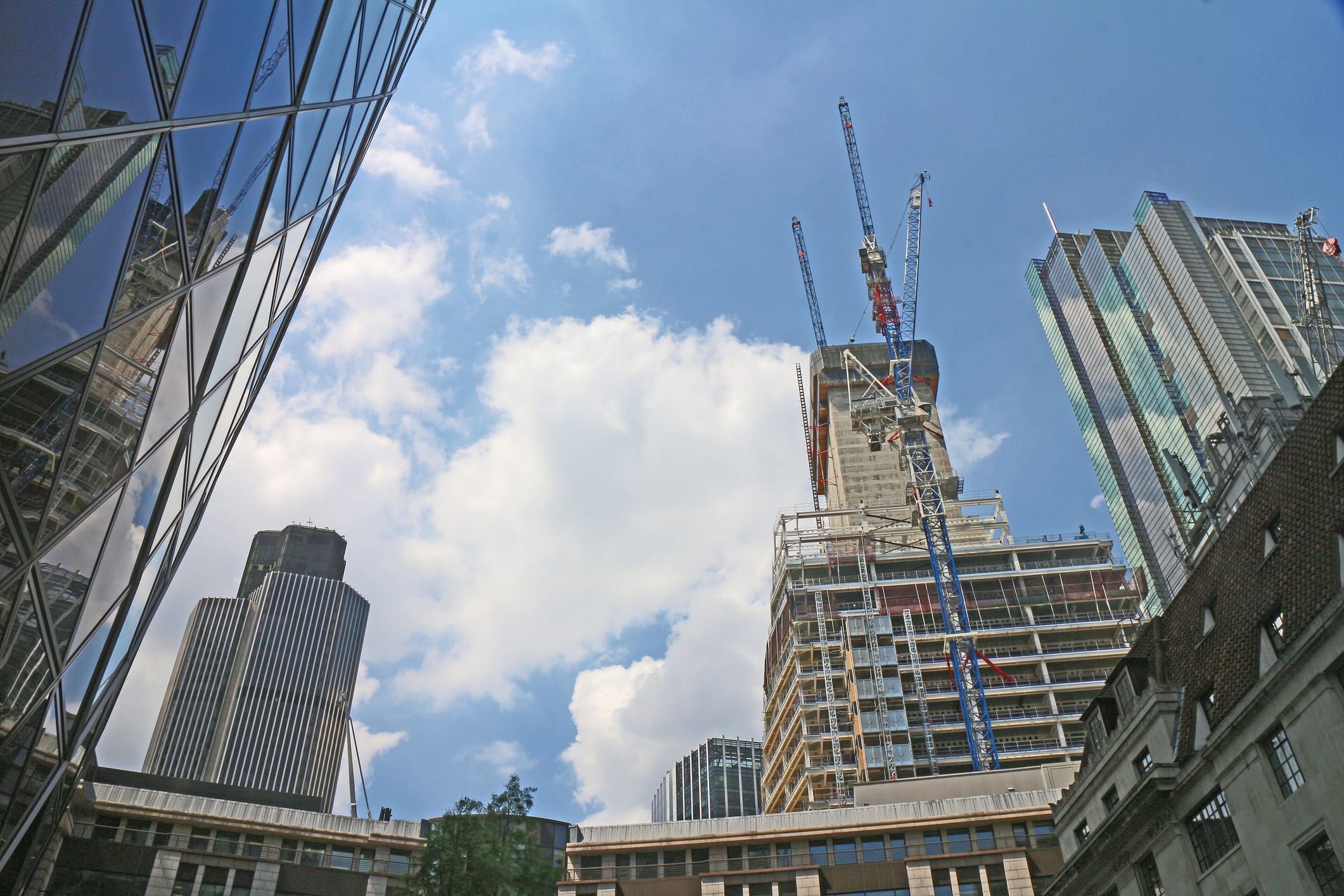
THE SCIENCE OF QUANTIK™
Publications
We publish insights through our LinkedIn newsletter, titled “The science of Quantik”, which are light bites of information covering news and insights relating to the construction industry and quantity surveying.
LinkedIn